Hello, engineers! This is Hermes Solution.
Today, we are going to discuss the safety assessment of AI/ML systems, which have become a major topic in the automotive industry. Specifically, we will explore how functional safety standards such as ISO 26262 can be integrated with AI/ML technology and what factors need to be considered to ensure safety.
Understanding AI and ML in the Automotive Industry
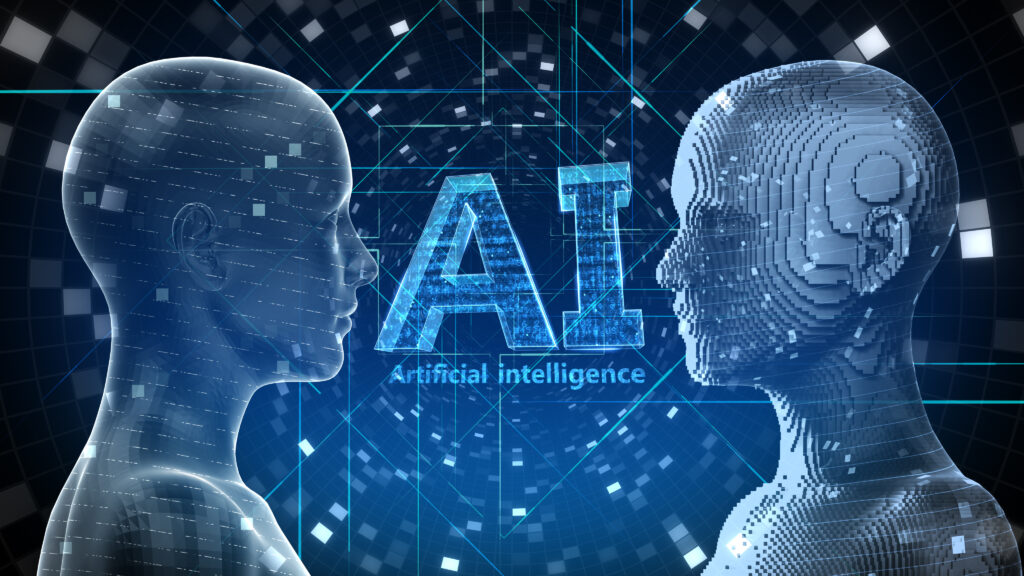
AI (Artificial Intelligence) and ML (Machine Learning) are technologies that analyze data, identify patterns, and make decisions autonomously. They have been widely adopted in the automotive sector. AI mimics human cognitive processes to solve complex problems, while ML, as a subset of AI, learns from data to enhance predictions and automate processes. These technologies contribute to the advancement of various automotive functions, including autonomous driving and ADAS (Advanced Driver Assistance Systems).
With the rapid advancement of the automotive industry, the role of Electronic Control Units (ECUs) and sensors has expanded, increasing the importance of semiconductor chips (e.g., SoC, MCU, ASIC). These semiconductor chips are crucial not only for vehicle performance but also for safety. As autonomous driving and ADAS technologies evolve, their significance grows even further. However, failures in automotive semiconductors can lead to critical driving safety issues, making functional safety standards essential for effective management and assessment.
ISO 26262 Part 11 focuses on ensuring safety in semiconductor design and development, supporting the development of more reliable automotive semiconductors.
Limitations of Applying ISO 26262 to AI/ML Systems
ISO 26262 is primarily designed for traditional hardware and software systems. However, AI/ML systems operate on data-driven learning and non-deterministic behavior, requiring a different assessment approach. Unlike conventional code-based systems, AI/ML models generate results based on learned data, making it difficult to predict outcomes in untrained environments. Additionally, AI/ML models may produce inconsistent results even with the same input, and their verification is inherently more complex than deterministic software systems.
To evaluate the safety of AI models and ensure compliance with functional safety standards, new verification methodologies must be adopted. Ensuring the safety of AI/ML systems requires rigorous data quality management, reliable dataset construction, and bias reduction. Furthermore, real-time data monitoring, uncertainty quantification, and functional safety integration should be key considerations.
ISO/PAS 8800: A New Standard for AI System Safety
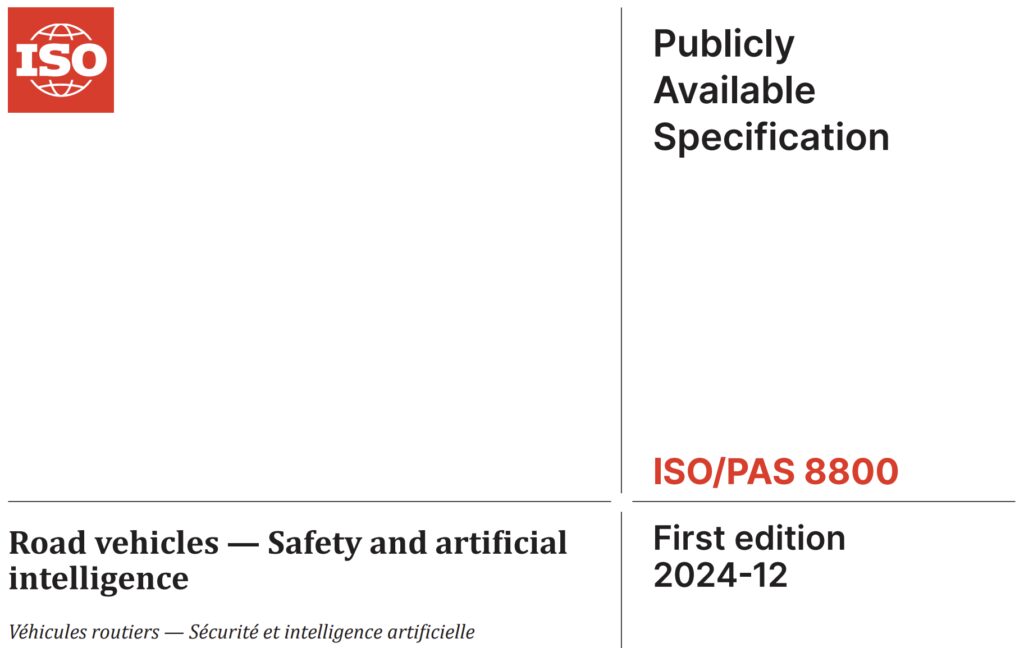
ISO/PAS 8800 is a newly developed standard designed to accommodate the unique characteristics of AI systems. It extends the principles of ISO 26262 and provides guidelines to ensure AI system safety. This standard aligns with ISO 21448 (SOTIF – Safety of the Intended Functionality) to assess functional insufficiencies in AI systems.
Key aspects of ISO/PAS 8800:
Ensures data quality and reliability for AI-based safety systems
Proposes evaluation criteria tailored to AI-driven safety applications
Strengthens trust in AI models by considering their data-dependent nature
ISO/PAS 8800 presents a more advanced safety assessment methodology compared to traditional functional safety approaches, helping to enhance the reliability of AI-based automotive systems.
A New Paradigm for AI System Safety Assessment
Ensuring the safety of AI-based systems requires a shift from conventional verification methods to new approaches that can objectively assess AI model reliability.
Key Approaches for AI Safety Assessment:
Developing new safety metrics – Establishing objective evaluation criteria for AI decision-making.
Continuous verification and improvement – Implementing an iterative process to enhance AI model robustness.
Explainability and transparency – Ensuring AI decisions can be interpreted and validated.
AI-based Hazard Analysis and Risk Assessment (HARA) can be leveraged to automatically detect hazards and enhance risk assessments through data-driven analysis. AI-powered risk analysis enables precise identification of potential hazards within a vehicle, improving overall safety evaluation.
Through real-time data analytics, AI can assess severity, exposure frequency, and controllability with greater accuracy, enabling a more reliable safety assessment framework.
Future Research Directions and Challenges
AI/ML systems must adapt and extend the existing evaluation criteria of ISO 26262. This requires incorporating data quality, model uncertainty, and learning algorithm characteristics into safety assessments.
Further studies should compare traditional HARA methods with AI-driven HARA approaches to validate AI’s strengths in risk assessment and measure its effectiveness. Additionally, real-world applications of ISO/PAS 8800 in AI/ML-based automotive systems should be analyzed to identify implementation challenges and areas for improvement.
A balanced approach is essential to ensure that AI systems deliver both innovation and safety. Moreover, new verification methodologies must be developed to assess whether AI models comply with ISO 26262 requirements.
By applying AI-driven risk analysis techniques, safety in various driving scenarios can be validated, ensuring greater trust and accuracy in AI/ML-based functional safety assessments.
Conclusion: Achieving Functional Safety in AI/ML-Driven Automotive Systems
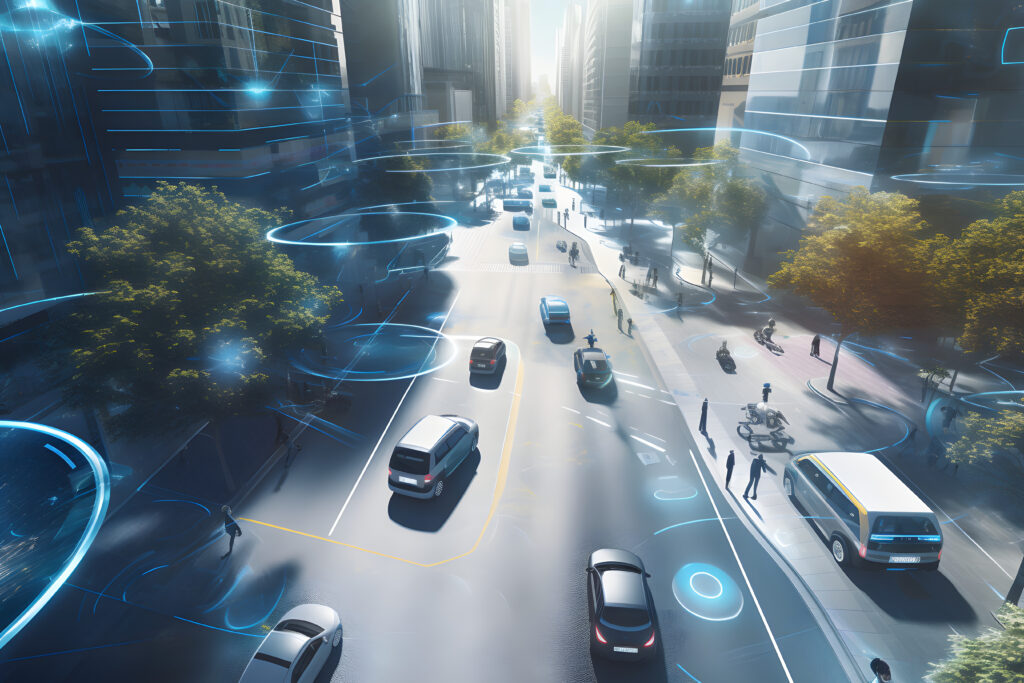
As AI/ML technologies become increasingly integral to the automotive industry, harmonizing them with functional safety standards is more critical than ever.
ISO 26262 has effectively ensured functional safety in traditional automotive systems, but AI/ML systems require a more flexible and extended approach. ISO/PAS 8800 addresses these challenges by providing a structured framework to enhance AI-based safety system reliability.
With the continuous advancement of semiconductor design and automotive technologies, securing functional safety for AI/ML-driven systems is a key requirement for the future of mobility.
At Hermes Solution, we provide expert consulting and support for ISO 26262 and AI/ML-based automotive system safety. Together, we can build a safer and more reliable mobility ecosystem.